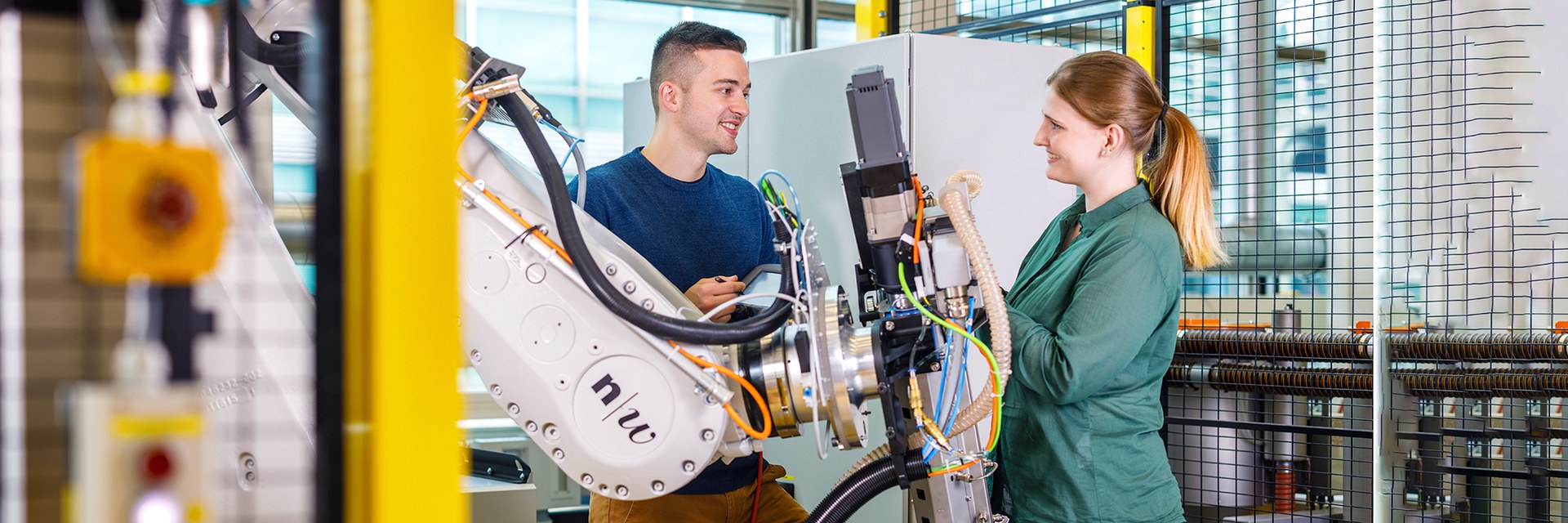
Analysis of the states of consciousness in the brain
By means of data analysis and machine learning, a Systems Engineering student has examined the characteristics of consciousness at the cerebral level.
To anaesthetise a patient for an operation, an anaesthetist must determine the dose of anaesthetic to be administered. However, safely determining the depth of anaesthesia is not possible with the standard monitoring systems currently in use. It can sometimes be the case therefore that full anaesthesia is not achieved for a patient, which can result in the patient having recollections of the operation, thereby sustaining significant psychosomatic damage. This phenomenon is referred to as "intraoperative awareness" and must be prevented.
The brain activity of different test persons at several different depths of anaesthesia from light to deep anaesthesia during sevoflurane anaesthesia was analysed in the form of EEG values and categorised into two statuses: "aware" and "anaesthesia". Furthermore, a comparison was made between classification methods that can form a model using these values. On this basis it is possible to determine the state of consciousness of new patients.
Four different cases may occur when classifying the data: "correct negative", "corrective positive", "false negative" and "false positive". In particular, the "false negative" classification case must be minimised in order to prevent intraoperative awareness. The best classification results were achieved using the "support vector machine" method while further sub-dividing the test person data. The rate of misclassification was reduced to 9.4% in this way.
Project information
- Client: The "Klinikum rechts der Isar" hospital, Technical University of Munich
- Implementation: Melanie Brügger
- Supervision: Professor Dr. Marcel Steiner-Curtis